- Published on
Python import data, indexing, slicing
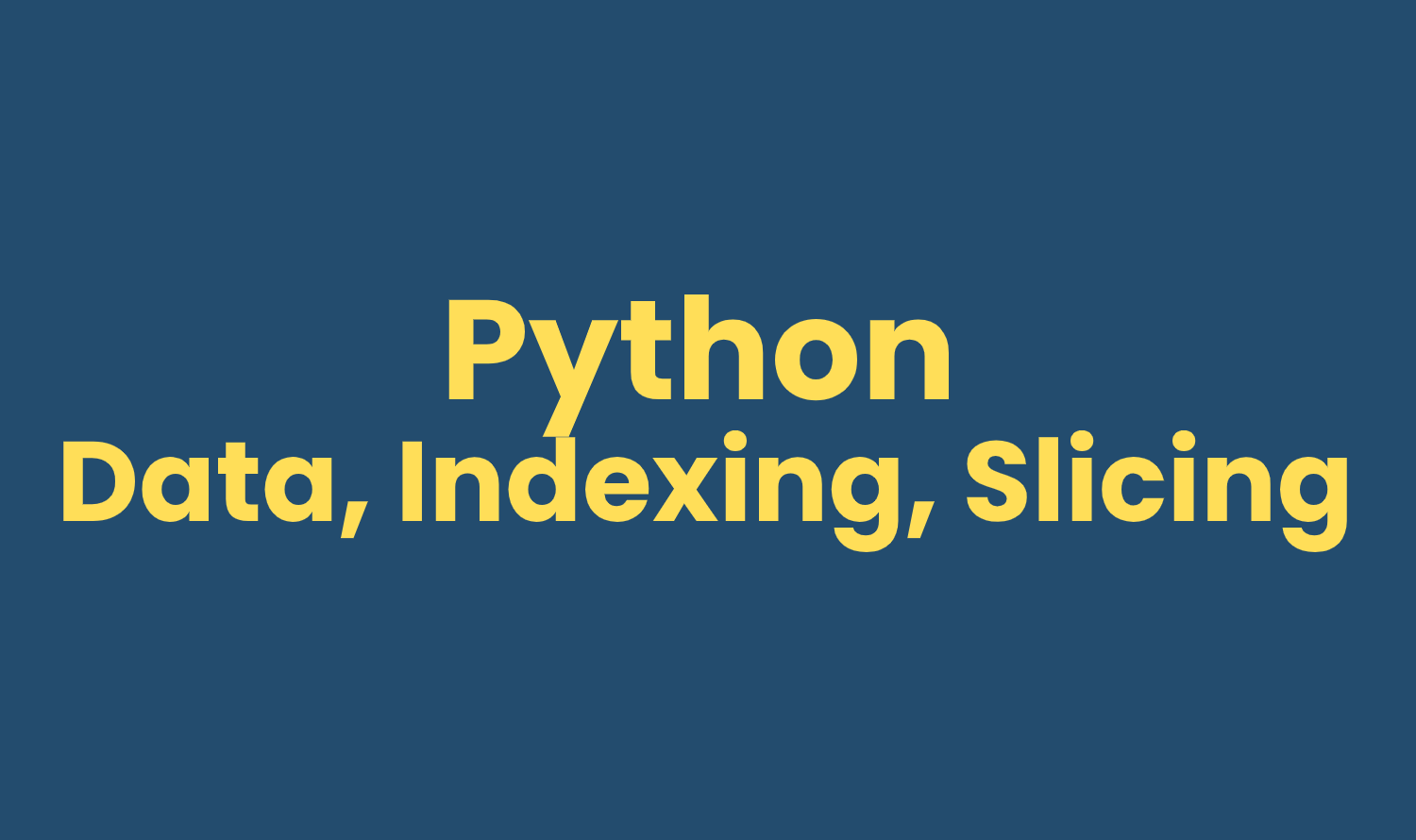
#Related Topics:
Common Shortcut
Select cell and press: Ctrl-Enter for run selected cells
- Alt-Enter for run cell and insert below
- A for insert new cell above selected cell
- B for insert new cell below selected cell
- M for make selected cell as markdown
Install Packages
pip install package_name
conda install package_name
use pip in command prompt
conda in Anaconda prompt
Working Directory
python
%pwd #pwd- Print Working Directory, will give you current working directory
text
'C:\\Users\\faisal\\Desktop\\Python\\Lesson-1'
Change directory
python
%cd C:\Users\faisal\Desktop\Python\Lesson-1 #cd- change directory
text
[WinError 2] The system cannot find the file specified: 'C:\\Users\\faisa\\Desktop\\Python\\Lesson-1 #cd- change directory'C:\Users\faisa\Desktop\Python\Lesson-1
Load Packages
python
import numpy as npimport pandas as pdimport pyodbc #require for sql server connection
Import csv from Local Machine
python
df=pd.read_csv('cost_of_living.csv') #press shift Tab to check all available parameter#df=pd.read_csv('c:\\Users\\faisa\\Desktop\\Python\\Lesson-1\\cost_of_living.csv',header=none)#df=pd.read_csv(r'c:\Users\faisa\Desktop\Python\Lesson-1\cost_of_living.csv')
python
df.head()
text
Rank City Cost of Living Index Rent Index \0 1 Hamilton, Bermuda 145.43 110.871 2 Zurich, Switzerland 141.25 66.142 3 Geneva, Switzerland 134.83 71.703 4 Basel, Switzerland 130.68 49.684 5 Bern, Switzerland 128.03 43.57Cost of Living Plus Rent Index Groceries Index Restaurant Price Index \0 128.76 143.47 158.751 105.03 149.86 135.762 104.38 138.98 129.743 91.61 127.54 127.224 87.30 132.70 119.48Local Purchasing Power Index0 112.261 142.702 130.963 139.014 112.71
Output CSV
python
df.to_csv('mydf.csv',index=False) #Don't forget to add '.csv' at the end.#df.to_csv(r'c:\Users\faisa\Desktop\Python\Lesson-1\my_df.csv',header=True,index=False) #Don't forget to add '.csv' at the end.#df.to_csv ('C:\\Users\\faisa\\Desktop\\Python\\Lesson-1\\my_df.csv', header=True,index=False) #Don't forget to add '.csv' at the end.
Import xlsx from Local Machine
python
df_exl=pd.read_excel('cost_of_living_xl.xlsx', sheet_name='sheet1') #specify sheet name from your excel file
Output Excel
python
df_exl.to_excel('mydf.xlsx',sheet_name='Sheet1')
Import from SQL Server
python
cnxn = pyodbc.connect("Driver={SQL Server};""Server=DESKTOP-H3MCNFQ;""Database=mydb;")# "uid=User;pwd=password")df_sql = pd.read_sql_query('select * from [cost_of_living_2018]', cnxn)
python
df_sql.head()
text
Rank City Cost_of_Living_Index Rent_Index \0 1 Hamilton, Bermuda 145.43 110.871 2 Zurich, Switzerland 141.25 66.142 3 Geneva, Switzerland 134.83 71.703 4 Basel, Switzerland 130.68 49.684 5 Bern, Switzerland 128.03 43.57Cost_of_Living_Plus_Rent_Index Groceries_Index Restaurant_Price_Index \0 128.76 143.47 158.751 105.03 149.86 135.762 104.38 138.98 129.743 91.61 127.54 127.224 87.30 132.70 119.48Local_Purchasing_Power_Index0 112.261 142.702 130.963 139.014 112.71
Import html Table
may need to install htmllib5,lxml, and BeautifulSoup4 packages:
conda install lxml
conda install html5lib
conda install BeautifulSoup4
python
df_html=pd.read_html('https://www.contextures.com/xlSampleData01.html',header=0)
python
df_html[0].head()
text
OrderDate Region Rep Item Units UnitCost Total0 1/6/2018 East Jones Pencil 95 1.99 189.051 1/23/2018 Central Kivell Binder 50 19.99 999.502 2/9/2018 Central Jardine Pencil 36 4.99 179.643 2/26/2018 Central Gill Pen 27 19.99 539.734 3/15/2018 West Sorvino Pencil 56 2.99 167.44
Import Remote Data
python
df_git = pd.read_csv('https://raw.githubusercontent.com/cs109/2014_data/master/mtcars.csv')df_git.head()
text
Unnamed: 0 mpg cyl disp hp drat wt qsec vs am gear \0 Mazda RX4 21.0 6 160.0 110 3.90 2.620 16.46 0 1 41 Mazda RX4 Wag 21.0 6 160.0 110 3.90 2.875 17.02 0 1 42 Datsun 710 22.8 4 108.0 93 3.85 2.320 18.61 1 1 43 Hornet 4 Drive 21.4 6 258.0 110 3.08 3.215 19.44 1 0 34 Hornet Sportabout 18.7 8 360.0 175 3.15 3.440 17.02 0 0 3carb0 41 42 13 14 2
python
df_git.to_csv ('C:\\Users\\faisa\\Desktop\\Python\\Lesson-1\\my_df_git.csv', header=True) #Don't forget to add '.csv' at the end.
Basic Information
python
df.shape
text
(538, 8)
python
df.columns
text
Index(['Rank', 'City', 'Cost of Living Index', 'Rent Index','Cost of Living Plus Rent Index', 'Groceries Index','Restaurant Price Index', 'Local Purchasing Power Index'],dtype='object')
python
df.info()
text
<class 'pandas.core.frame.DataFrame'>RangeIndex: 538 entries, 0 to 537Data columns (total 8 columns):Rank 538 non-null int64City 538 non-null objectCost of Living Index 538 non-null float64Rent Index 538 non-null float64Cost of Living Plus Rent Index 538 non-null float64Groceries Index 538 non-null float64Restaurant Price Index 538 non-null float64Local Purchasing Power Index 538 non-null float64dtypes: float64(6), int64(1), object(1)memory usage: 33.7+ KB
python
df.count()
text
Rank 538City 538Cost of Living Index 538Rent Index 538Cost of Living Plus Rent Index 538Groceries Index 538Restaurant Price Index 538Local Purchasing Power Index 538dtype: int64
python
df.sum()
text
Rank 144991City Hamilton, BermudaZurich, SwitzerlandGeneva, Sw...Cost of Living Index 34220Rent Index 14624.4Cost of Living Plus Rent Index 24769.5Groceries Index 32062.1Restaurant Price Index 31733.7Local Purchasing Power Index 48515.9dtype: object
python
df.min()
text
Rank 1City Aachen, GermanyCost of Living Index 20.86Rent Index 3.82Cost of Living Plus Rent Index 13.26Groceries Index 19.26Restaurant Price Index 12.06Local Purchasing Power Index 1.88dtype: object
python
df.max()
text
Rank 538City Zurich, SwitzerlandCost of Living Index 145.43Rent Index 115.36Cost of Living Plus Rent Index 128.76Groceries Index 149.86Restaurant Price Index 158.75Local Purchasing Power Index 168.93dtype: object
python
df.describe()
text
Rank Cost of Living Index Rent Index \count 538.000000 538.000000 538.000000mean 269.500000 63.605874 27.182937std 155.451493 21.359530 17.207302min 1.000000 20.860000 3.82000025% 135.250000 46.060000 13.00250050% 269.500000 67.805000 25.09500075% 403.750000 78.430000 35.432500max 538.000000 145.430000 115.360000Cost of Living Plus Rent Index Groceries Index \count 538.000000 538.000000mean 46.039944 59.594926std 18.330342 22.168789min 13.260000 19.26000025% 30.997500 40.47750050% 48.030000 61.63000075% 58.005000 74.362500max 128.760000 149.860000Restaurant Price Index Local Purchasing Power Indexcount 538.000000 538.000000mean 58.984498 90.178271std 26.243787 36.637241min 12.060000 1.88000025% 34.490000 58.08750050% 64.065000 95.16000075% 77.165000 120.140000max 158.750000 168.930000
python
df.mean()
text
Rank 269.500000Cost of Living Index 63.605874Rent Index 27.182937Cost of Living Plus Rent Index 46.039944Groceries Index 59.594926Restaurant Price Index 58.984498Local Purchasing Power Index 90.178271dtype: float64
python
df.median()
text
Rank 269.500Cost of Living Index 67.805Rent Index 25.095Cost of Living Plus Rent Index 48.030Groceries Index 61.630Restaurant Price Index 64.065Local Purchasing Power Index 95.160dtype: float64
python
#df.isna() #will return True or False for each value, if null then True, if not null then Falsedf.isna().sum() #will return total number of null for each column
text
Rank 0City 0Cost of Living Index 0Rent Index 0Cost of Living Plus Rent Index 0Groceries Index 0Restaurant Price Index 0Local Purchasing Power Index 0dtype: int64
Basic Indexing and Selecting and Slicing
python
df['City']
text
0 Hamilton, Bermuda1 Zurich, Switzerland2 Geneva, Switzerland3 Basel, Switzerland4 Bern, Switzerland5 Lausanne, Switzerland6 Reykjavik, Iceland7 Stavanger, Norway8 Lugano, Switzerland9 Oslo, Norway10 Trondheim, Norway11 Bergen, Norway12 Kyoto, Japan13 New York, NY, United States14 Nassau, Bahamas15 San Francisco, CA, United States16 Copenhagen, Denmark17 Luxembourg, Luxembourg18 Anchorage, AK, United States19 Honolulu, HI, United States20 Tokyo, Japan21 Brooklyn, NY, United States22 Paris, France23 Limerick, Ireland24 Rockville, MD, United States25 Bloomington, IN, United States26 Washington, DC, United States27 Arhus, Denmark28 Singapore, Singapore29 Aalborg, Denmark...508 Lahore, Pakistan509 Pristina, Kosovo (Disputed Territory)510 Chandigarh, India511 Ahmedabad, India512 Surat, India513 Chennai, India514 Goa, India515 Indore, India516 Kolkata, India517 Lucknow (Lakhnau), India518 Kiev, Ukraine519 Jaipur, India520 Karachi, Pakistan521 Hyderabad, India522 Cairo, Egypt523 Dnipro, Ukraine524 Nagpur, India525 Bhopal, India526 Vadodara, India527 Mangalore, India528 Lviv, Ukraine529 Mysore, India530 Bhubaneswar, India531 Kharkiv, Ukraine532 Visakhapatnam, India533 Kochi, India534 Coimbatore, India535 Alexandria, Egypt536 Navi Mumbai, India537 Thiruvananthapuram, IndiaName: City, Length: 538, dtype: object
python
df[['City','Restaurant Price Index']]
text
City Restaurant Price Index0 Hamilton, Bermuda 158.751 Zurich, Switzerland 135.762 Geneva, Switzerland 129.743 Basel, Switzerland 127.224 Bern, Switzerland 119.485 Lausanne, Switzerland 132.126 Reykjavik, Iceland 133.197 Stavanger, Norway 143.548 Lugano, Switzerland 122.309 Oslo, Norway 124.0910 Trondheim, Norway 134.7611 Bergen, Norway 119.6112 Kyoto, Japan 54.5913 New York, NY, United States 100.0014 Nassau, Bahamas 104.1715 San Francisco, CA, United States 91.0616 Copenhagen, Denmark 121.2317 Luxembourg, Luxembourg 109.6118 Anchorage, AK, United States 84.5519 Honolulu, HI, United States 82.8620 Tokyo, Japan 58.9321 Brooklyn, NY, United States 100.5822 Paris, France 91.7723 Limerick, Ireland 82.9324 Rockville, MD, United States 74.7425 Bloomington, IN, United States 75.4326 Washington, DC, United States 85.0027 Arhus, Denmark 102.8228 Singapore, Singapore 64.4029 Aalborg, Denmark 101.14.. ... ...508 Lahore, Pakistan 26.39509 Pristina, Kosovo (Disputed Territory) 22.78510 Chandigarh, India 20.18511 Ahmedabad, India 20.13512 Surat, India 19.84513 Chennai, India 18.26514 Goa, India 22.96515 Indore, India 17.77516 Kolkata, India 23.18517 Lucknow (Lakhnau), India 18.76518 Kiev, Ukraine 22.01519 Jaipur, India 18.48520 Karachi, Pakistan 21.62521 Hyderabad, India 18.93522 Cairo, Egypt 22.55523 Dnipro, Ukraine 22.74524 Nagpur, India 18.73525 Bhopal, India 16.21526 Vadodara, India 16.02527 Mangalore, India 16.04528 Lviv, Ukraine 17.88529 Mysore, India 13.31530 Bhubaneswar, India 14.91531 Kharkiv, Ukraine 18.44532 Visakhapatnam, India 18.07533 Kochi, India 13.94534 Coimbatore, India 15.21535 Alexandria, Egypt 17.66536 Navi Mumbai, India 14.14537 Thiruvananthapuram, India 12.06[538 rows x 2 columns]
python
df[2:10] #specific rows, all columns
text
Rank City Cost of Living Index Rent Index \2 3 Geneva, Switzerland 134.83 71.703 4 Basel, Switzerland 130.68 49.684 5 Bern, Switzerland 128.03 43.575 6 Lausanne, Switzerland 127.50 52.326 7 Reykjavik, Iceland 123.78 57.257 8 Stavanger, Norway 118.61 39.838 9 Lugano, Switzerland 118.24 52.919 10 Oslo, Norway 117.23 49.28Cost of Living Plus Rent Index Groceries Index Restaurant Price Index \2 104.38 138.98 129.743 91.61 127.54 127.224 87.30 132.70 119.485 91.24 126.59 132.126 91.70 118.15 133.197 80.61 106.09 143.548 86.73 117.74 122.309 84.46 112.42 124.09Local Purchasing Power Index2 130.963 139.014 112.715 127.956 88.957 118.148 119.869 102.94
python
#.at labels baseddf.at[3,'Rent Index']
text
49.68
python
#.iat integer baseddf.iat[3,3]
text
49.68
python
df.head(10)
text
Rank City Cost of Living Index Rent Index \0 1 Hamilton, Bermuda 145.43 110.871 2 Zurich, Switzerland 141.25 66.142 3 Geneva, Switzerland 134.83 71.703 4 Basel, Switzerland 130.68 49.684 5 Bern, Switzerland 128.03 43.575 6 Lausanne, Switzerland 127.50 52.326 7 Reykjavik, Iceland 123.78 57.257 8 Stavanger, Norway 118.61 39.838 9 Lugano, Switzerland 118.24 52.919 10 Oslo, Norway 117.23 49.28Cost of Living Plus Rent Index Groceries Index Restaurant Price Index \0 128.76 143.47 158.751 105.03 149.86 135.762 104.38 138.98 129.743 91.61 127.54 127.224 87.30 132.70 119.485 91.24 126.59 132.126 91.70 118.15 133.197 80.61 106.09 143.548 86.73 117.74 122.309 84.46 112.42 124.09Local Purchasing Power Index0 112.261 142.702 130.963 139.014 112.715 127.956 88.957 118.148 119.869 102.94
python
#loc is label based
python
#select specific rows and columndf.loc[:,['City', 'Cost of Living Index', 'Rent Index','Cost of Living Plus Rent Index', 'Groceries Index','Restaurant Price Index', 'Local Purchasing Power Index']]
text
City Cost of Living Index Rent Index \0 Hamilton, Bermuda 145.43 110.871 Zurich, Switzerland 141.25 66.142 Geneva, Switzerland 134.83 71.703 Basel, Switzerland 130.68 49.684 Bern, Switzerland 128.03 43.575 Lausanne, Switzerland 127.50 52.326 Reykjavik, Iceland 123.78 57.257 Stavanger, Norway 118.61 39.838 Lugano, Switzerland 118.24 52.919 Oslo, Norway 117.23 49.2810 Trondheim, Norway 114.22 42.3911 Bergen, Norway 112.31 40.3012 Kyoto, Japan 100.33 24.5813 New York, NY, United States 100.00 100.0014 Nassau, Bahamas 99.73 40.4515 San Francisco, CA, United States 97.84 115.3616 Copenhagen, Denmark 97.62 50.6617 Luxembourg, Luxembourg 95.37 61.5918 Anchorage, AK, United States 94.99 40.1219 Honolulu, HI, United States 94.15 62.8220 Tokyo, Japan 93.81 37.0721 Brooklyn, NY, United States 93.79 76.2422 Paris, France 92.87 50.3023 Limerick, Ireland 92.73 27.7124 Rockville, MD, United States 92.66 64.0025 Bloomington, IN, United States 92.14 33.6426 Washington, DC, United States 91.94 73.3027 Arhus, Denmark 91.90 34.8228 Singapore, Singapore 91.40 71.8929 Aalborg, Denmark 91.17 26.81.. ... ... ...508 Lahore, Pakistan 29.53 6.67509 Pristina, Kosovo (Disputed Territory) 29.25 9.38510 Chandigarh, India 29.04 6.47511 Ahmedabad, India 28.67 6.24512 Surat, India 28.66 4.69513 Chennai, India 28.42 7.12514 Goa, India 28.30 8.27515 Indore, India 28.06 4.66516 Kolkata, India 27.99 7.77517 Lucknow (Lakhnau), India 27.55 4.90518 Kiev, Ukraine 27.52 12.43519 Jaipur, India 27.11 5.19520 Karachi, Pakistan 27.10 7.46521 Hyderabad, India 26.92 6.89522 Cairo, Egypt 26.49 5.43523 Dnipro, Ukraine 26.39 6.63524 Nagpur, India 26.23 4.96525 Bhopal, India 26.07 4.13526 Vadodara, India 25.59 4.01527 Mangalore, India 25.46 5.70528 Lviv, Ukraine 25.31 8.10529 Mysore, India 25.20 4.01530 Bhubaneswar, India 24.89 4.68531 Kharkiv, Ukraine 24.85 8.29532 Visakhapatnam, India 24.66 4.85533 Kochi, India 24.65 6.31534 Coimbatore, India 24.61 5.35535 Alexandria, Egypt 23.78 4.34536 Navi Mumbai, India 23.44 6.25537 Thiruvananthapuram, India 20.86 5.10Cost of Living Plus Rent Index Groceries Index Restaurant Price Index \0 128.76 143.47 158.751 105.03 149.86 135.762 104.38 138.98 129.743 91.61 127.54 127.224 87.30 132.70 119.485 91.24 126.59 132.126 91.70 118.15 133.197 80.61 106.09 143.548 86.73 117.74 122.309 84.46 112.42 124.0910 79.58 103.50 134.7611 77.58 101.79 119.6112 63.80 118.44 54.5913 100.00 100.00 100.0014 71.14 85.34 104.1715 106.29 107.52 91.0616 74.97 77.53 121.2317 79.08 82.71 109.6118 68.53 101.18 84.5519 79.04 104.69 82.8620 66.45 99.67 58.9321 85.33 92.73 100.5822 72.34 87.29 91.7723 61.37 87.15 82.9324 78.84 87.76 74.7425 63.93 112.83 75.4326 82.95 92.74 85.0027 64.37 71.50 102.8228 81.99 83.64 64.4029 60.13 73.79 101.14.. ... ... ...508 18.50 26.83 26.39509 19.67 25.97 22.78510 18.15 29.40 20.18511 17.85 31.42 20.13512 17.10 31.97 19.84513 18.14 31.17 18.26514 18.64 29.80 22.96515 16.78 27.74 17.77516 18.24 28.53 23.18517 16.62 27.25 18.76518 20.24 21.96 22.01519 16.54 27.65 18.48520 17.63 25.60 21.62521 17.26 27.60 18.93522 16.33 23.23 22.55523 16.86 20.46 22.74524 15.97 26.55 18.73525 15.49 22.49 16.21526 15.18 27.85 16.02527 15.93 26.85 16.04528 17.01 20.50 17.88529 14.98 29.39 13.31530 15.14 28.22 14.91531 16.87 19.26 18.44532 15.11 25.83 18.07533 15.80 26.93 13.94534 15.32 25.23 15.21535 14.40 23.19 17.66536 15.15 24.02 14.14537 13.26 21.98 12.06Local Purchasing Power Index0 112.261 142.702 130.963 139.014 112.715 127.956 88.957 118.148 119.869 102.9410 108.2911 99.2912 77.9213 100.0014 58.6915 92.9616 113.3117 127.4218 124.9219 103.0820 106.4221 87.0422 97.6223 93.9324 130.7925 96.9226 120.6227 109.4728 95.8929 106.35.. ...508 51.44509 64.57510 68.83511 73.59512 57.84513 72.34514 54.55515 50.42516 56.30517 76.10518 37.48519 76.50520 39.06521 80.90522 25.27523 31.06524 95.19525 66.21526 80.63527 94.53528 26.88529 42.49530 57.56531 27.19532 63.97533 77.70534 53.23535 23.75536 111.99537 66.25[538 rows x 7 columns]
python
#select all rows but specific columndf.loc[[0,3],['City', 'Cost of Living Index', 'Rent Index','Cost of Living Plus Rent Index', 'Groceries Index','Restaurant Price Index', 'Local Purchasing Power Index']]
text
City Cost of Living Index Rent Index \0 Hamilton, Bermuda 145.43 110.873 Basel, Switzerland 130.68 49.68Cost of Living Plus Rent Index Groceries Index Restaurant Price Index \0 128.76 143.47 158.753 91.61 127.54 127.22Local Purchasing Power Index0 112.263 139.01
python
#iloc is integer based
python
#Specific rows but all columns, remember here last index number is excludingdf.iloc[:5]
Rank City Cost of Living Index Rent Index \0 1 Hamilton, Bermuda 145.43 110.871 2 Zurich, Switzerland 141.25 66.142 3 Geneva, Switzerland 134.83 71.703 4 Basel, Switzerland 130.68 49.684 5 Bern, Switzerland 128.03 43.57Cost of Living Plus Rent Index Groceries Index Restaurant Price Index \0 128.76 143.47 158.751 105.03 149.86 135.762 104.38 138.98 129.743 91.61 127.54 127.224 87.30 132.70 119.48Local Purchasing Power Index0 112.261 142.702 130.963 139.014 112.71
python
#Select all rows and specific column, remember here last index number is excludingdf.iloc[:,2:5]
text
Cost of Living Index Rent Index Cost of Living Plus Rent Index0 145.43 110.87 128.761 141.25 66.14 105.032 134.83 71.70 104.383 130.68 49.68 91.614 128.03 43.57 87.305 127.50 52.32 91.246 123.78 57.25 91.707 118.61 39.83 80.618 118.24 52.91 86.739 117.23 49.28 84.4610 114.22 42.39 79.5811 112.31 40.30 77.5812 100.33 24.58 63.8013 100.00 100.00 100.0014 99.73 40.45 71.1415 97.84 115.36 106.2916 97.62 50.66 74.9717 95.37 61.59 79.0818 94.99 40.12 68.5319 94.15 62.82 79.0420 93.81 37.07 66.4521 93.79 76.24 85.3322 92.87 50.30 72.3423 92.73 27.71 61.3724 92.66 64.00 78.8425 92.14 33.64 63.9326 91.94 73.30 82.9527 91.90 34.82 64.3728 91.40 71.89 81.9929 91.17 26.81 60.13.. ... ... ...508 29.53 6.67 18.50509 29.25 9.38 19.67510 29.04 6.47 18.15511 28.67 6.24 17.85512 28.66 4.69 17.10513 28.42 7.12 18.14514 28.30 8.27 18.64515 28.06 4.66 16.78516 27.99 7.77 18.24517 27.55 4.90 16.62518 27.52 12.43 20.24519 27.11 5.19 16.54520 27.10 7.46 17.63521 26.92 6.89 17.26522 26.49 5.43 16.33523 26.39 6.63 16.86524 26.23 4.96 15.97525 26.07 4.13 15.49526 25.59 4.01 15.18527 25.46 5.70 15.93528 25.31 8.10 17.01529 25.20 4.01 14.98530 24.89 4.68 15.14531 24.85 8.29 16.87532 24.66 4.85 15.11533 24.65 6.31 15.80534 24.61 5.35 15.32535 23.78 4.34 14.40536 23.44 6.25 15.15537 20.86 5.10 13.26[538 rows x 3 columns]